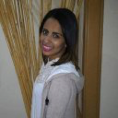
Fannia Pacheco MontillaPhD Student in Computer Science
- LIUPPA
LIUPPA
UFR des Sciences et Techniques de la Côte basqueAllée du Parc Montaury64600 Angletf.pacheco @ univ-pau.fr
Parcours
Professional Experience
2015-2016 |
Researcher at GIDTEC-Mechanical Engineering Department, Universidad Politécnica Salesiana, Ecuador |
Oct 2012 - |
Researcher at CEMiSiD- Universidad de Los Andes, Venezuela Knowledge extraction from a chemical process in an oil industry, by using data mining |
Nov 2014 - |
Hired Professor at Universidad de Los Andes, Mérida, Venezuela |
Sep 2012- | Hired Professor at Universidad Nacional Experimental Politécnica de la Fuerza Armada Nacional, Mérida, Venezuela |
Education
July 2015 |
Magister Scientiae in Computer Science, Universidad de Los Andes, Mérida, Venezuela |
Dec 2012 | Undergraduate Degree in Systems Engineer Control and Automation, Universidad de Los Andes, Mérida, Venezuela Thesis: Design, construction and control of an exoskeleton oriented to rehabilitate the index finger of the hand |
Thèmes de recherche
- Machine Learning
- Internet traffic
- Autonomic computing
Encadrement
Thesis
Title
Classification techniques for the management of the “Quality of Service” in satellite communication systems
Description
Traffic analysis stands for all the strategies intended to find relationships, patterns, anomalies, and misconfigurations, among others things, in Internet traffic; particularly, traffic classification is a subgroup of strategies in this field. Recently, traffic classification has become a challenging task due to the widespread of new technologies, such as traffic encryption and encapsulation, which intricate or unable classical traffic classification strategies. Machine Learning (ML) emerges as a new direction in this field, showing signs of future success, such as knowledge extraction from encrypted traffic and more accurate Quality of Service management. In the present work, we study the whole process needed to perform traffic analysis with ML. We identified the main problems found in the literature as well as in experimental tests in order to define some strategies to fill these gaps. The ML process starts by extracting information from historical data, several features that characterize the problem are computed and used to build ML models that can classify or predict the status of the case study. One of the main obstacles, for applying ML in this domain, is the unavailability of knowledge base that serves as primordial resource for building ML solutions. This knowledge base is set up with a variety of traffic that defines the interactions between the Internet network actors. In this sense, we propose a cloud platform in order to emulate and to record Internet traffic; moreover, this platform will allow defining an experimental setup for testing the ML solutions. Following, the features extracted from the Internet network play a key factor for the success of the ML models. We study several approaches for performing feature extraction, where the statistical based features seem to be the most effective ones. Considering that these features suffer from some drawbacks related to the distribution of the traffic type (streaming, web content, etc), we present a novel approach to perform the feature extraction process. Another additional challenging task found was the dynamicity of the Internet network leading to rapid obsolescence of the ML solutions. This problem is faced with an incremental learning approach that can help to increase the base knowledge acquired, and to update the ML models regularly. Each of the previous steps will be part of a main architecture that will handle the traffic classification in an autonomic way. This autonomic system aims at adapting and configuring itself given the current status of the network.
Members
Thesis Director |